PyPEER Integration¶
PEER is a previously developed support vector regression-based method for retrospectively estimating eye gaze from the fMRI signal in the eye’s orbit. PyPEER is the Python package created by Jake Son [https://github.com/ChildMindInstitute/PyPEER] which implements the PEER method in Python.
C-PAC can prepare your pipeline results directly for Predictive Eye Estimation, given the appropriate input files. The PyPEER package comes pre-installed in the C-PAC Docker and Singularity containers, so no setup is necessary.
Configuring CPAC to Run PyPEER¶
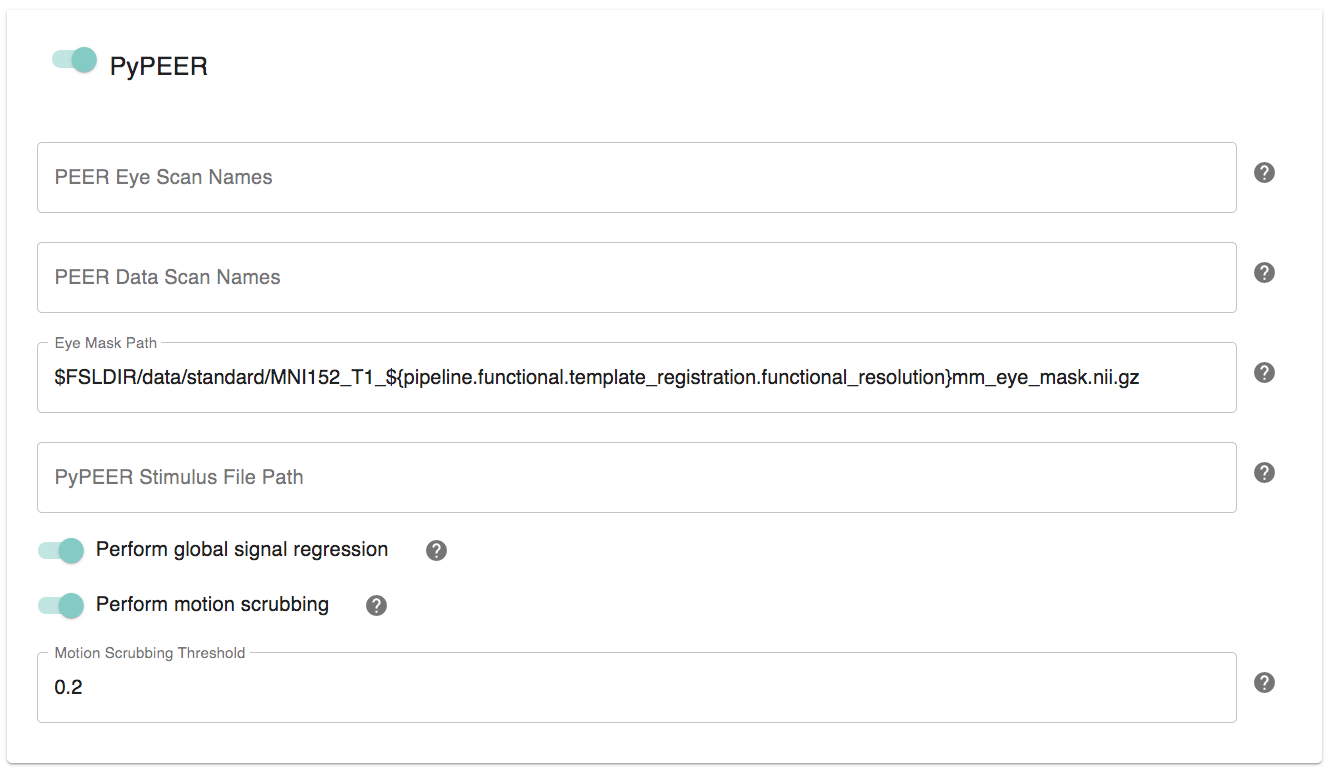
PEER Eye Scan Names: Scan/series names of the PEER calibration scans to be used for training the model.
PEER Data Scan Names: Scan/series names of the naturalistic viewing data to be used for estimation.
Eye Mask Path: Path of the file of a template-space eye mask. The default selection is bundled with the C-PAC container from the FSL installation.
PyPEER Stimulus File Path: This is a file describing the stimulus locations from the calibration sequence.
Perform Global Signal Regression: PyPEER employs a minimal preprocessing strategy. If global signal regression is desired, this can be selected here. C-PAC will not employ its standard nuisance regression selections on scans selected for PEER calibration and estimation.
Perform Motion Scrubbing: PyPEER employs a minimal preprocessing strategy. If motion scrubbing is desired, this can be selected here. C-PAC will not employ its standard nuisance regression selections on scans selected for PEER calibration and estimation.
Motion Scrubbing Threshold: The threshold for motion (in mm) to use for motion scrubbing, if it is selected. PyPEER employs the Power (2012) measurement for framewise displacement.
Configuration Without the GUI¶
The following nested key/value pairs will be set to these defaults if not defined in your pipeline configuration YAML.
PyPEER:
# Training of eye-estimation models. Commonly used for movies data/naturalistic viewing.
run: Off
# PEER scan names to use for training
# Example: ['peer_run-1', 'peer_run-2']
eye_scan_names: []
# Naturalistic viewing data scan names to use for eye estimation
# Example: ['movieDM']
data_scan_names: []
# Template-space eye mask
eye_mask_path: $FSLDIR/data/standard/MNI152_T1_${func_resolution}_eye_mask.nii.gz
# PyPEER Stimulus File Path
# This is a file describing the stimulus locations from the calibration sequence.
stimulus_path: None
minimal_nuisance_correction:
# PyPEER Minimal nuisance regression
# Note: PyPEER employs minimal preprocessing - these choices do not reflect what runs in the main pipeline.
# PyPEER uses non-nuisance-regressed data from the main pipeline.
# Global signal regression (PyPEER only)
peer_gsr: True
# Motion scrubbing (PyPEER only)
peer_scrub: False
# Motion scrubbing threshold (PyPEER only)
scrub_thresh: 0.2
References¶
Jake Son, Lei Ai, Ryan Lim, Ting Xu, Stanley Colcombe, Alexandre Rosa Franco, Jessica Cloud, Stephen LaConte, Jonathan Lisinski, Arno Klein, R. Cameron Craddock, Michael Milham. Evaluating fMRI-Based Estimation of Eye Gaze during Naturalistic Viewing. https://doi.org/10.1101/347765
Fox, M.D., Zhang, D., Snyder, A.Z., Raichle, M.E., 2009. The global signal and observed anticorrelated resting state brain networks. J Neurophysiol 101, 3270-3283.
Power, J.D., Barnes, K.A., Snyder, A.Z., Schlaggar, B.L., Petersen, S.E., 2011. Spurious but systematic correlations in functional connectivity MRI networks arise from subject motion. Neuroimage 59, 2142-2154.
Power, J.D., Barnes, K.A., Snyder, A.Z., Schlaggar, B.L., Petersen, S.E., 2012. Steps toward optimizing motion artifact removal in functional connectivity MRI; a reply to Carp. Neuroimage.