Longitudinal Preprocessing¶
Background¶
Longitudinal preprocessing pipeline is designed to process longitudinal data with two or more sessions. Based on Reuter et al. 2012, all secions of subjects are first preprocessed independently and then a within-subject template is created by averaging all sessions of the subject. Subsequently the within-subject template is registered to standard space and all sections are then registered to the longitudinal template in standard space.
Configuring CPAC to Run Longitudinal Preprocessing Pipeline¶
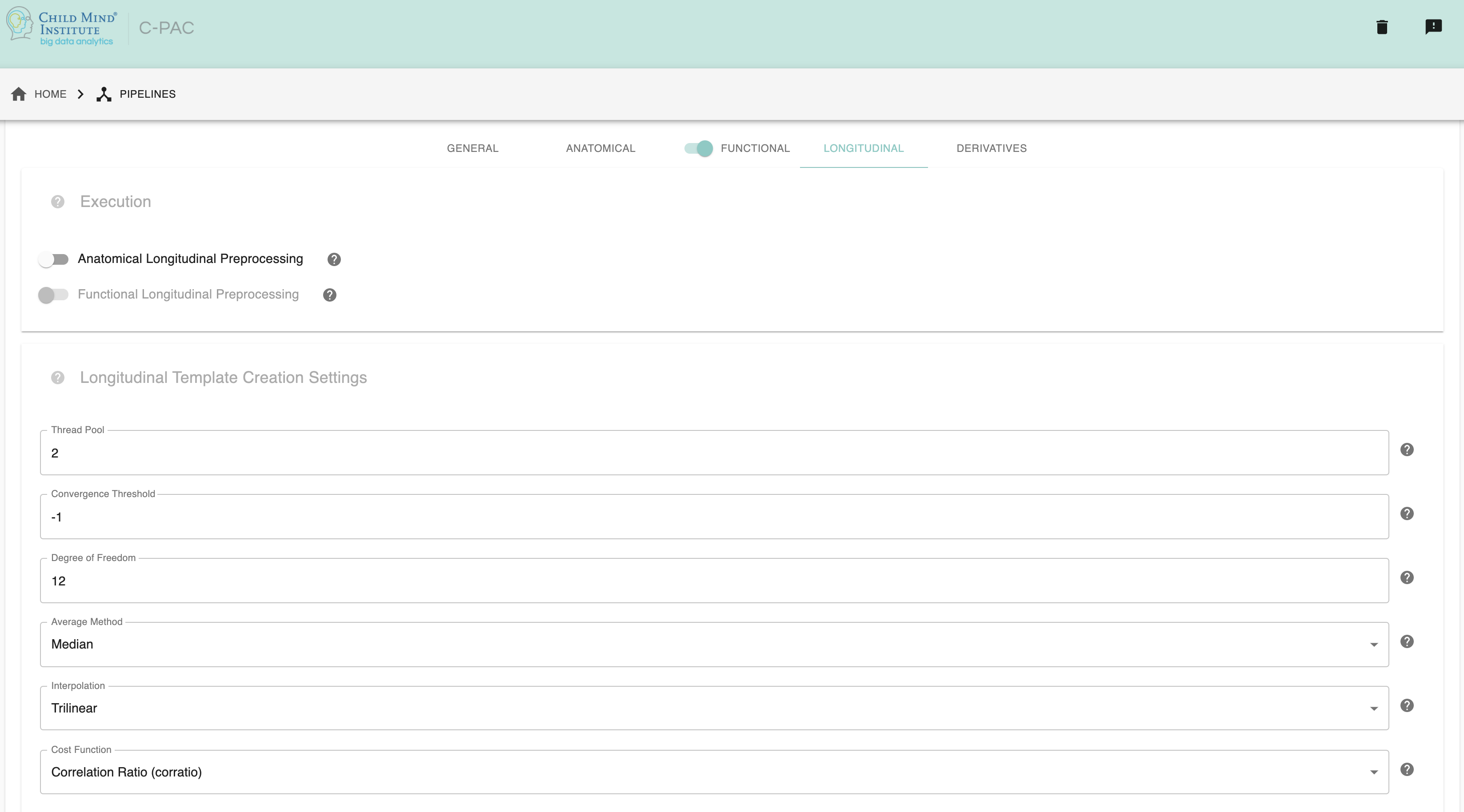
Longitudinal - [On, Off]: Run longitudinal preprocessing pipeline or not. Default is Off.
Average Method - [string]: Method to average longitudinal template. Default is median.
DOF - [integer]: Transform degree of freedom in flirt. Default is 12.
Interpolation - [string]: Interpolation in flirt. Default is trilinear.
Cost Function - [string]: Cost function in flirt. Default is corratio.
Thread Pool - [integer]: Number of threads in a thread pool. More threads can speed up the longitudinal template creation process. Default is 2.
Convergence Threshold - [integer]: Convergence threshold of longitudinal template. Default is -1, which uses numpy.finfo(np.float64).eps.
Configuration Without the GUI¶
The following nested key/value pairs will be set to these defaults if not defined in your pipeline configuration YAML.
longitudinal_template_generation:
# If you have multiple T1w's, you can generate your own run-specific custom
# T1w template to serve as an intermediate to the standard template for
# anatomical registration.
# This runs before the main pipeline as it requires multiple T1w sessions
# at once.
run: Off
# Freesurfer longitudinal template algorithm using FSL FLIRT
# Method to average the dataset at each iteration of the template creation
# Options: median, mean or std
average_method: median
# Degree of freedom for FLIRT in the template creation
# Options: 12 (affine), 9 (traditional), 7 (global rescale) or 6 (rigid body)
dof: 12
# Interpolation parameter for FLIRT in the template creation
# Options: trilinear, nearestneighbour, sinc or spline
interp: trilinear
# Cost function for FLIRT in the template creation
# Options: corratio, mutualinfo, normmi, normcorr, leastsq, labeldiff or bbr
cost: corratio
# Number of threads used for one run of the template generation algorithm
thread_pool: 2
# Threshold of transformation distance to consider that the loop converged
# (-1 means numpy.finfo(np.float64).eps and is the default)
convergence_threshold: -1
References¶
Martin Reuter, Nicholas J.Schmansky, H. Diana Rosas, Bruce Fischl. Within-subject template estimation for unbiased longitudinal image analysis. Neuroimage. 2012 July 16; 61(4): 1402–1418. doi:10.1016/j.neuroimage.2012.02.084.